Computational Histology
Analysis of histology images of the human tissue biopsies remains the most reliable way of diagnosing and grading cancer. The current gold-standard for quantification and diagnostics based on prepared slides of human tissue is often a human expert looking through a microscope at the slides.
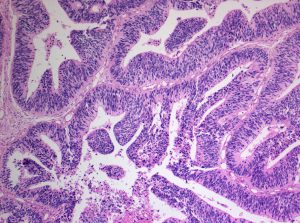
Our aim is to enhance and extend this expensive and time-consuming process by performing automated analysis of high-resolution images of tissue. This investigation involves designing a combination of algorithms, scanners, stains and biomarkers that allow fast and accurate identification of aspects of clinical interest.
Recent Publications
-
Yue, X, Dimitriou, N, Caie, P, Harrison, D & Arandjelovic, O 2019, Colorectal cancer outcome prediction from H&E whole slide images using machine learning and automatically inferred phenotype profiles. in O Eulenstein, H Al-Mubaid & Q Ding (eds), Proceedings of 11th International Conference on Bioinformatics and Computational Biology, BICOB 2019: Honolulu; United States; 18 March 2019 through 20 March 2019. EPiC Series in Computing, vol. 60, EasyChair, pp. 139-127, 11th
International Conference on Bioinformatics and Computational Biology (BICOB), Honolulu, United States, 18/03/19. https://doi.org/10.29007/n912
Dimitriou, N, Arandjelović, O & Caie, PD 2019, ‘Deep learning for whole slide image analysis: an overview‘, Frontiers in Medicine, vol. 6, 264. https://doi.org/10.3389/fmed.2019.00264